Bioinformatics Hackathon award winners
Lyda Hill Award
This award judged the best project overall for technical merit, innovation, clinical application, feasibility, and wow factor.
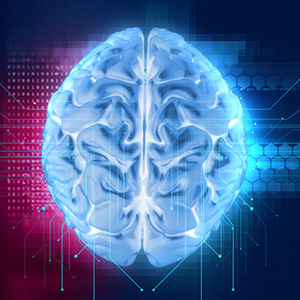
A team led by UT Southwestern Bioinformatics Core Facility staff and clinical fellows at Children’s Medical Center Dallas used machine learning to predict risk of neurologic injury in pediatric extracorporeal membrane oxygenation (ECMO) patients. The most likely neurologic injury for these patients is stroke, so the team built a framework to statistically assess actionable events that either lead to stroke or improve outcomes. The team achieved 93 percent accuracy in predicting a brain bleed in this patient population using the machine-learning model they designed and tested. This tool could lead to the first meaningful changes in ECMO patient neurological outcomes in decades.
Team leads: Dr. Neel Shah and Dr. Abdelaziz Farhat, Pediatric Critical Care Fellows
Best Use of Visualization
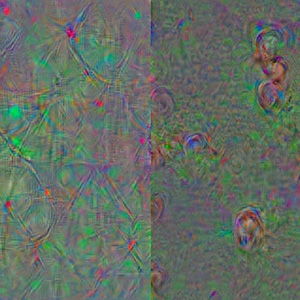
This team assembled a software called RU-Meta that uses data from four-hour-long movies of stage 3 melanoma biopsies to predict the potential of these tumors to either progress to highly metastatic stage 4 or remain stagnant. Leveraging deep learning neural networks to identify meaningful behavioral patterns of cells in these biopsies, the software achieved 92 percent prediction accuracy. An open-source software tool called DeepDream was then used to probe what the trained network “thinks” is distinguishing between cells with high and low metastatic potential. These “dream images” revealed that highly metastatic cells create long spiculated extensions across time-projected images, while low metastatic cells behave like compact spiral/nautilus-like objects. The visualization of these abstract behaviors, which escape any human observer, garnered the team the award.
Team lead: UTSW scientific programmer Dr. Andrew Jamieson
Most Out-of-the Box Award: Highest Innovation Score
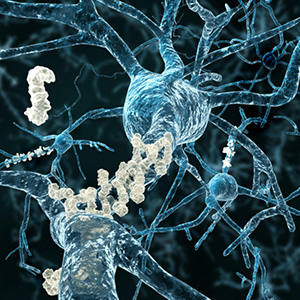
This team set out to simulate molecular dynamics of potential drug target regions. The ras family of G-proteins is one of the most important and universally mutated proteins in cancer. But due to its small size and other variables, it is currently undruggable by any direct means. The team provided a 20-microsecond-long all-atom molecular dynamics simulation trajectory of H-Ras at thermal equilibrium under physiological conditions. The goal was to identify the shape and location of transient pockets that may be stabilized through binding of targeted molecules and to correlate the emergence of such pockets with structural changes in the protein active site.
Team lead: Dr. Milo Lin, Assistant Professor in the Cecil H. and Ida Green Comprehensive Center for Molecular, Computational, and Systems Biology and a Cecil H. and Ida Green Endowed Scholar in Biomedical Computational Science
Best Desk-to-Bedside Award: Highest Clinical Application Score
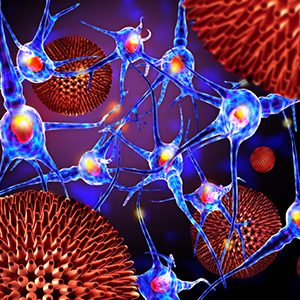
Mobilizing a patient’s own immune system to kill tumor cells is the current frontier in cancer treatment. At the same time, the early response of the immune system to the presence of a tumor in a patient can potentially become a powerful tool for cancer diagnosis. The design of such a reporter system hinges on identifying the specialized adaptive areas of T-cell surface receptors that control the interaction of these immune cells with cells of a particular tumor. This team aimed to develop a machine-learning method to classify the adaptive receptor areas from about 20,000 receptor sequences of T-cells that have interacted with tumors and from about 10,000 receptor sequences of T-cells without interaction. Moving forward, this classifier may be able to detect the presence of tumors based on T-cell surface receptor sequences excised from patients with unknown cancer disease status.
Team lead: Dr. Bo Li, Assistant Professor of Bioinformatics and Immunology
Most Likely to Become a Startup Award: Highest Feasibility Score
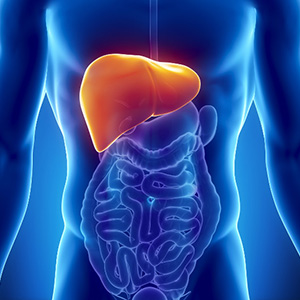
This project was related to determining an algorithm for staging of liver disease. Fatty liver disease progresses though several stages and the diagnosis of the stage is instrumental to treatment and prognosis. The McDonald lab has devolved a lipidomics platform using mass spectroscopy along with shotgun lipidomics to measure thousands of known and unknown lipids easily collected via a plasma sample taken from a patient. The focus of this project was to develop a machine-learning algorithm using plasma lipid data to provide staging for a patient’s liver disease diagnosis. The data consists of about 20 de-identified samples for each of the diagnosis stages with the ground truth known from the core liver punch biopsy.
Team lead: Dr. Jeffrey McDonald, Associate Professor of Molecular Genetics and in the Center for Human Nutrition
Next Sci-Fi Blockbuster Award: Highest Wow Factor Score
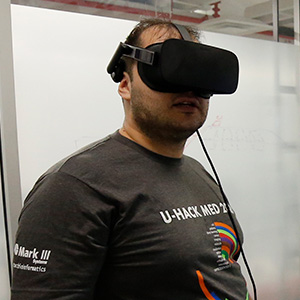
This project focused on developing deep learning (DL) models in virtual reality. DL is a popular subfield of machine learning that has demonstrated significant advances. DL is particularly useful in supervised learning, and especially so in computer vision. Recent technical advances in virtual reality have enabled the development of highly immersive and interactive experiences. The specific idea was to build a “shelf” of components commonly used in DL, such as convolutional layers, pooling layers, and fully connected layers. After designing a network as such, the user can press a virtual button to run the data through and start the back-propagation algorithm that trains the model. For images, one can watch the intermediate layers during learning with the simple gesture of grabbing them and pulling them, making it possible for novice machine learners to understand the action of these tools.
Team lead: Dr. Murat Can Çobanoğlu, a Distinguished Fellow in Bioinformatics